Next:Entropy
of a ContinuousUp:Entropy
Rate and Entropy
Previous:Entropy
Rate Go to:Table
of Contents
Entropy Series
This subsection deals with a measure of entropy commonly referred
as entropy series, where in the probability distribution we have
.
This quantity is given by
where
with
and
.
Let
and
be two sequences. Then it can easily be checked that the series
diverges and the series
converges. Let
be the sum of the series. Consider
,
then
In view of the fact that the series
diverges, we get
as infinite. In order that the entropy series converges, we need some restrictions.
If there is a convergent series of positive terms
such that
also converges. Then by use of the inequality (1.9), we get the following
bound:
The following properties give better restrictions to bound the entropy
series
.
Property 1.64. If for some
,
then
Property 1.65. For each nondecreasing probability sequence
i.e.,
,
the entropy series
converges iff the series
converges.
Property 1.66. For each nondecreasing sequence
,
the following bound on the entropy series holds:
where
is a nonnegative, nondecreasing sequence of numbers with the property that
for some
,
and
Property 1.67. For each nondecreasing probability sequence
,
the following bound on the entropy series hold:
where
is as given in property 1.65.
Property 1.68. (Maximum-Entropy Principle). The entropy
series
under the constraints
-
(a)
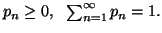
-
(b)

is maximized when
and the maximum value is given by
where
are normalizing constants.
Note 1.6. Using the condition
,
we get
|
|
|
(1.12) |
By applying the conditions
,
we can calculate the constants
.
The probability distribution (1.12) is famous in the literature as ``Gibbs
distribution''.
Particular cases: The property 1.69 admits the following interesting
particular cases.
-
(i) The probability distribution
,
that maximizes the corresponding entropy
is the uniform distribution given by
.
-
(ii) The probability distribution
,
that maximizes the corresponding entropy series
subject to the constraint
is the geometric distribution given by
, where b can be obtained by using the given constraints.
-
(iii) The probability distribution
,
(integers)
that maximizes the corresponding entropy
subject to the constraints
and
is the discrete normal distribution given by
,
where
can be obtained by using the given constraints.
Property 1.69. Let
be a sequence of real numbers with the property that for some
,
then the following bound on the entropy series hold:
Property 1.70. The following bound on the entropy series holds:
where
is as given in (1.8).
The following properties are also worth emphasizing, whose details can
be seen in Capocelli et al. (1988a;b)[25]
[26].
Property 1.71. The following bound on the entropy series hold:
where
is the Riemann zeta function and
is the unique solution of the equation
and
is defined as
when
is a power of a prime p; 0 otherwise.
Property 1.72. The following bound on the entropy series hold:
where
is a bounded function of
,
with
Property 1.73. For each nonincreasing probability distribution
and for each
,
the following bound on the entropy series holds:
where
is a constant independent of the probability distribution
and for
,
,
we have
and
Property 1.74. For each nonincreasing probability distribution
,
the following bound on the entropy series holds:
where
Property 1.75. For each nonincreasing probability distribution
and for each
,
the following limits hold:
-
(i)

-
(ii)

-
(iii)

-
(iv)

where
and
Note 1.7. The good references for the sections 1.4, 1.5, 1.6
and 1.7 are the book by Csiszár and Körner (1981) [30],
Guiasu (1977) [41], McEliece (1977) [72],
etc..
21-06-2001
Inder Jeet Taneja
Departamento de Matemática - UFSC
88.040-900 Florianópolis, SC - Brazil